BBC Headlined: The AI industry could use as much energy as the Netherlands. While there are several examples of how AI could play an important role in lowering the environmental impact of human activities. For example, by improving climate models, the creation of AI smart homes which reduce household CO2 emissions by up to 40% or the reduction in transportation waste. There are also concerns about AI’s Energy Usage.
Data centres, “The physical homes”, where many AI models like GPT-3, BERT, LaMDA etc. are trained and deployed are known to be energy intensive. In numbers, these data centres account for roughly 1 to 2% of the world’s total electricity usage. These data centres are not only power hungry but also thirsty, with Google, Microsoft and Meta collectively withdrawing an estimated 2.2 billion cubic meters of water in 2022. To give an idea of the scale of these numbers, it is equivalent to the total annual water usage of Denmark a deux.
This article explains how AI models affect the environment, focusing on their carbon and water footprints. It aims to help you understand the energy AI models use, so you can make informed choices about using AI in a way that balances benefits with sustainability. We will also provide 5 practical tips for saving energy when using AI and show you how to calculate your own AI carbon footprint.
Did you know that a single ChatGPT question consumes more energy than a Google Search? The energy consumption of a Google search is around 0.0003 kWh. A ChatGPT-4 interaction can consume anywhere from 0.001 to 0.01 kWh, depending on the model size and tokens used. That means a single GPT interaction uses 15 times more energy than a Google search. To put the numbers in perspective, a 60W light bulb uses up 0.06kWh in an hour.
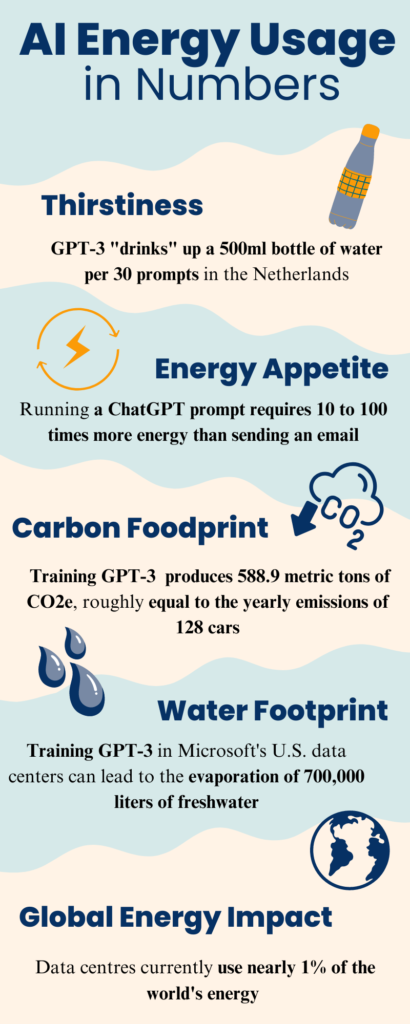
AI and Energy Consumption
Artificial Intelligence (AI) refers to a range of technologies and methods that enable machines to exhibit intelligent behaviour. One of these methods is Generative AI (Gen AI), which is used to create new content, such as text, images, or videos. For instance, ChatGPT is a text tool, while OpenAI’s DALL-E is a tool that turns text prompts into images. Although Gen AI have different applications, they share a common process: an initial training phase
followed by an inference phase
, where the model requires energy.
Training Phase
The training phase of AI models is often considered the most energy-intensive. In this stage, an AI model is fed large datasets. The model’s initially arbitrary parameters are adjusted to align the predicted output closely with the target output. This process results in learning to predict specific words or sentences based on a given context. Once deployed, these parameters direct the model’s behaviour.
The energy used in the training process is measured in megawatt-hours (MWh). To give context, the average household in the United States consumes approximately 10.7 MWh annually. Data on the training of Large Language Models (LLMs) such as BLOOM, GPT-3, Gopher and OPT reveals the amount of electricity consumed during training, with BLOOM consuming 433 MWh, GPT-3 using 1,287 MWh, Gopher using 1,066 MWh and OPT using 324 MWh. Before settling on the final model, there may have been multiple iterations of training runs.
“Where” to train a large AI model can significantly affect the environmental cost. For instance, the BLOOM model was trained in France, where 60% of their electricity comes from nuclear power. This allowed the carbon intensity of the BLOOM model to be very low, at 0.057 kg CO2e/kWh, compared to the average carbon intensity of the US, which is 0.387 kg CO2e/kWh. Therefore, although BLOOM consumed almost the same amount of energy in the training phase as Llama, the resulting emissions were only one-tenth the amount.
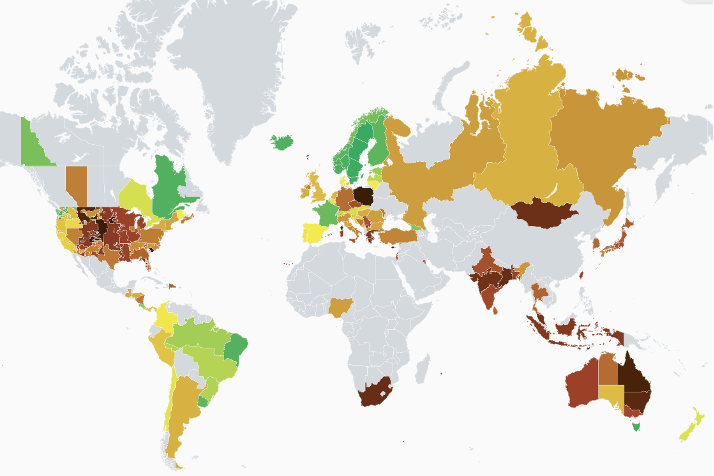
Inference Phase
Once the models are trained, they are used in production to generate outputs based on new data in the inference phase. In the case of ChatGPT, this involves creating responses to user prompts.
Although the models used to generate these responses consume a modest amount of energy per session. Alphabet, the parent company of Google, announced that the cost of using a Language Model (LLM) can be up to 10 times higher than that of a standard keyword search. A standard Google search consumes approximately 0.3 Wh of electricity, which suggests an electricity consumption of roughly 3 Wh per LLM interaction. ChatGPT responds to 195 million requests daily, requiring an average electricity consumption of 564 MWh per day (as of February 2023), or 2.9 Wh per request. Therefore, the energy demand during the inference phase appears to be significantly higher in comparison to the estimated 1,287 MWh used during a GPT-3’s training run.
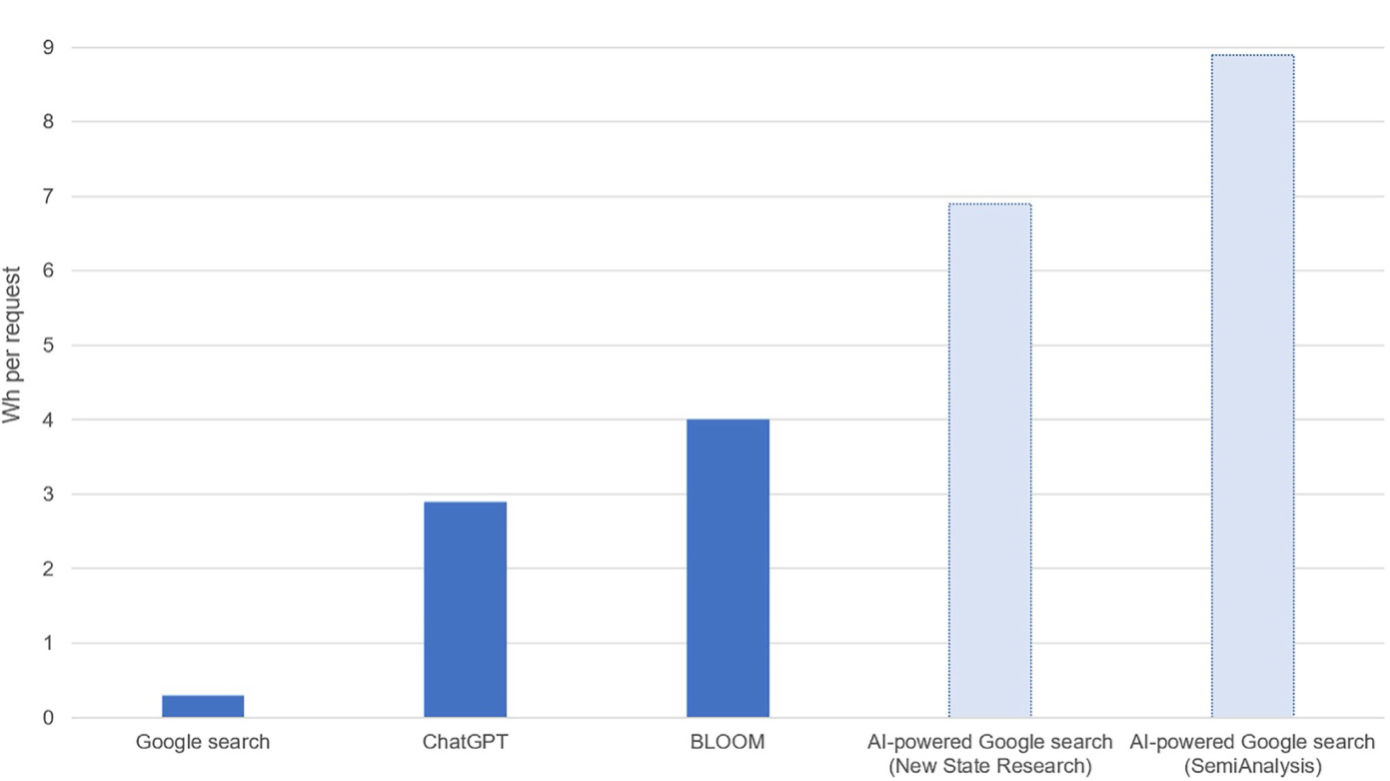
GPT-3’s Water Consumption Footprint
It is important not to focus solely on AI models’ energy usage and carbon footprint but also to consider the water footprint when examining their environmental impact. For instance, the process of training GPT-3 in Microsoft’s modern U.S. data centres can cause the evaporation of 700,000 litres of clean freshwater. When considering the larger picture, the global demand for AI could result in 4.2 to 6.6 billion cubic meters of water withdrawal by 2027. This figure is greater than the annual water withdrawal of 4 to 6 times that of Denmark or half of the United Kingdom.
The source article discusses OpenAI's GPT-3 model. This is possible due to Microsoft's transparency in disclosingWater Usage Effectiveness
(WUE) and Power Usage Effectiveness (PUE) data by location, which is not public information for other companies.
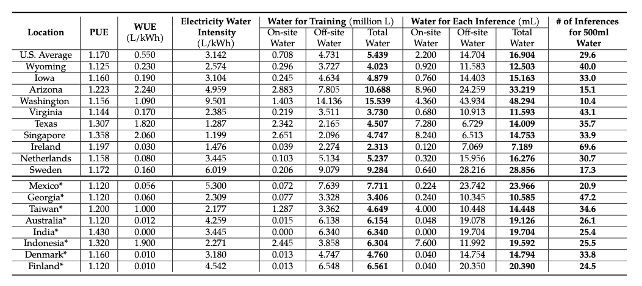
So, how thirsty is ChatGPT-3 when we use it? On average, in America, it uses 16.9 mL of water per prompt or interaction, which empties up a 500 mL water bottle after 29.6 instances. However, the thirstiness of ChatGPT-3 shows variation across regions. For example, in Ireland, it takes nearly 70 interactions to empty a 500 mL water bottle.
Trade-offs
In Sweden, the water bottle empties quickly (17.3 interactions), but this isn’t necessarily detrimental given the region’s abundant water resources. This highlights a potential trade-off: managing the balance between water and carbon footprints. Swedish data centers, leveraging an excess of water and strong renewable energy infrastructure, may consume more water but with a lower carbon impact.
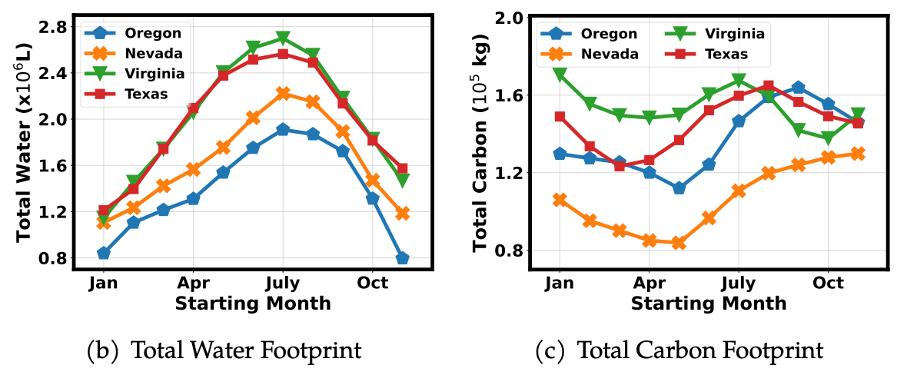
Some tips we discuss involve using something called an API. A programming tool that lets you build your own ChatGPT tool. It sounds difficult, but it isn’t. Check out this article, where we help you step-by-step through the process.
5 Tips to Reduce Your AI Environmental Footprint
- Question whether you need AI usage. Of course, the easiest way to reduce your AI environmental impact is to not use it anymore. However, start with being selective about whether your question or problem needs the use of GenAI. Maybe, a less energy-intensive solution, like a Google search is appropriate.
- Use a larger AI model only when it adds value. While larger model offers more capabilities, be mindful of their usage. Instead, adopt smaller models, when possible because they are more energy-efficient. For example, if you want to generate a short and simple text, such as a headline or a caption, reflect on whether it is necessary to use a large and complex model like ChatGPT-4.
- Reuse and Recycle AI Responses: When possible, reuse previous AI-generated outputs for similar prompts. ChatGPT remembers and stores responses. When you have a similar questions refer back to past responses. This can avoid unnecessary recomputation, thereby saving energy.
- Limit the output length of an AI model: The benefit of using an AI model like ChatGPT via an API is that you can limit the number of tokens (words and characters) the model generates to shorten responses. For instance, limit responses to 150 tokens for tasks where concise answers are sufficient. This reduces the computational effort and, consequently, the energy consumption.
To keep things concise when using the Desktop version of ChatGPT, you might try phrases like:
- “Can you summarize this briefly?”
- “Focus on the main idea, please.”
- “Keep it short and simple.”
Another useful statement in your prompt is to be concrete and the number of words the output length should be. For instance, you could say, “Explain what an API is in under 50 words,” to get a compact answer.
Here is a use case for the API:
from openai import OpenAI
client = OpenAI()
completion = client.chat.completions.create(
model="gpt-3.5-turbo",
messages=[
{"role": "user", "content": "tell a good joke in the form of a question. Do not yet give the answer."}
],
max_tokens= 150 # Limit the maximum tokens for the model's response
)
print(completion.choices[0].message)
Two birds with one stone: Thanks to the pay-for-use system of the API, conserving energy also translates in saving money.
5. Batch prompts: When you have several questions at once, or some tasks that aren’t immediately necessary, consider grouping your prompts. This process called, batching prompts, uses less computational resources.
Check out here an example case of the desktop version.
And again for the API:
from openai import OpenAI # Import the OpenAI package
import os # Import the os package
client = OpenAI(api_key=os.environ.get("OPENAI_API_KEY"))
completion = client.chat.completions.create(
model="gpt-3.5-turbo",
messages=[
{"role": "user", "content": "I want you to do the following things: Tell a good joke in the form of a question. Do not yet give the answer. Provide a brief summary of environmental tips. Explain the concept of batch processing in AI."}
]
)
print(completion.choices[0].message.content)
Get a vision of your Energy usage
While we have discussed the energy usage of ChatGPT, it is may still a bit vague and you may be still wondering, how much CO2 will I exactly omit. So if you’re curious about the actual impact of your energy usage when using ChatGPT. You can measure this impact directly using CodeCarbon. This is an open-source software package that integrates into a Python codebase (file) and tracks the amount of carbon dioxide (CO2) produced by the cloud or by local computing resources used to execute your code. This means, that if you are using the API it will state the CO2 impact of your prompt.
Here’s how to use CodeCarbon
:
Step 1: Install CodeCarbon
Open your command line interface and run:
pip install codecarbon
Step 2: Integrate CodeCarbon
with your Python Code:
from openai import OpenAI
import os
from codecarbon import EmissionsTracker
client = OpenAI(api_key= os.environ.get("OPENAI_API_KEY"))
# Set up the emissions tracker
tracker = EmissionsTracker()
tracker.start()
completion = client.chat.completions.create(
model="gpt-3.5-turbo",
messages=[
{"role": "user", "content": "I want you to do the following things: Tell a good joke in the form of a question. Do not yet give the answer. Provide a brief summary of environmental tips. Explain the concept of batch processing in AI."}
]
)
print(completion.choices[0].message.content)
# Stop the tracker and print the estimated emissions
emissions = tracker.stop()
print(f"Estimated CO2 emissions for this batch of AI tasks: {emissions} kg")
This script will track the emissions for the interactions you run through the API. This means you have an exact statement of your environmental impact.
Resources
- Li, P., Yang, J., Islam, M. A., & Ren, S. (2023). Making ai less” thirsty”: Uncovering and addressing the secret water footprint of ai models.
- Vries, The growing energy footprint of artificial intelligence, Joule (2023), https://doi.org/10.1016/ j.joule.2023.09.004