Welcome to the next part of our Prompt Engineering series. In the first two parts, we explored the Key Prompt Engineering Principles: asking detailed questions, using action verbs, iterating on prompts, and specifying distinct prompt components. You might have also followed our in-depth tutorial on the OpenAI API Python
package. So far, we have focused on engineering prompts to retrieve more targeted answers from the model. Now, we will take control of the response format. This part will focus on retrieving structured outputs.
Customizing Output
An important assumption when using Large Language Models, such as ChatGPT, is that they do not give structured output without being explicitly asked for it. Meaning, it is necessary to be specific in your response about what you want to receive. Just as asking detailed questions is vital (key principle 1
), specifying the desired output structure is equally important. This includes the length of the response in words, bullet points, or paragraphs. Additionally, If the output needs to be in a table, list, structured paragraphs, or another custom format, you must clearly indicate this.
Example Case: Tables
Let’s start with a simple example: retrieving a table as output by changing our prompt. When asking the model to generate a table, you should clearly mention the columns you expect. For instance, you can instruct the model to create a table with three examples of machine learning models, specifying columns for their name, description, and key characteristics:
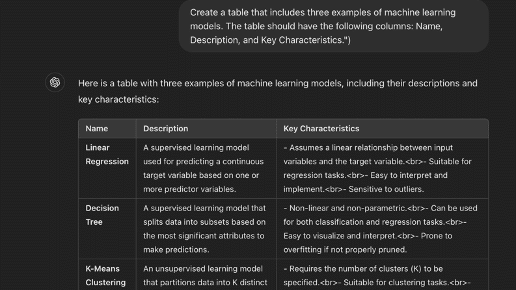
And indeed, we see how the output meets our requirements.
For those using the API, you can use the template provided in the previous article:
# Using the defined function in Article 2
response = ChatGPT_API("Create a table that includes three examples of machine learning models. The table should have the following columns: Name, Description, and Key Characteristics.")
print(response)
Example Case: Text Output
This functionality extends to text prompts as well. If you want to generate paragraphs with a specific structure, you should explicitly mention the format requirements in the prompt. For example, you can ask the model to generate a structured paragraph with clear headings and subheadings about the benefits of open-source research. Of course, this is not limited to chapter headers, you can always specify what subject will be in the chapters yourself.
Example prompt: “Create a structured paragraph that includes headings and subheadings, using a I – II – III structure, about the benefits of open-source research.“
The Benefits of Open Source Research
I. Enhanced Collaboration
Open source research fosters a collaborative environment where researchers from diverse backgrounds can contribute and share their expertise. This collective effort accelerates the pace of discovery and innovation. By allowing unrestricted access to research data and methodologies, open source research breaks down silos and encourages interdisciplinary collaboration, leading to more comprehensive and robust findings.
II. Increased Transparency and Reproducibility
Transparency is a cornerstone of scientific integrity. Open source research ensures that all aspects of a study, from data
...
Here, we see how the output is formatted with a main header: “The Benefits of Open Source Research” and subheadings: “I. Enhanced Collaboration,” “II. Increased Transparency and Reproducibility,”.
Constructing Custom Response Frameworks
Until now, we have let ChatGPT determine the content of our tables and paragraphs. Let’s take the next step by adjusting the prompt to retrieve custom response frameworks
. This can be best explained by examining an example:
Let’s say you are studying and you find some R code online that answers a coding subject you are curious about. You are looking for solutions in Python, but you are not quite comfortable with R.
We can obtain an explanation and transformation of the R
code into Python
, along with an explanation (as nothing is learned from just a simple transformation) in one prompt by breaking this process down into distinct parts. For example, we start by identifying the input coding language, then we request a transformation into Python
along with an explanation. We denote each part of the output with the <>
placeholders to clearly show what specific output needs to be included in the subparts.
Benefits Of Using Placeholders
- Organizing Information: Each placeholder defines a separate segment of the AI model’s response, ensuring that the information is systematically arranged. This makes the output clear and easy to follow, regardless of the context.
- Guiding the Model: The placeholders act as markers that guide the transformation process. They specify the necessary components of the response, whether it involves code, text, or other types of data. This directs the AI model in the right direction and prevents it from wandering off, resulting in more accurate and precise answers.
- Improving Understanding: In the example, we also requested the original code to be shown. This adds to the learning experience by not only displaying the transformed output but also assure that the model explains the transformation and the reasons behind it. This helps you develop a deeper understanding of the content.
- Template Filling: These placeholders, in the case of code transformation, can function as templates that can be filled with the appropriate content. Creating a file with these placeholders for standardized tasks, such as code explanation, transformation, or text translation. Now prompt engineering becomes a straightforward exercise. This makes the process of creating prompts efficient and consistent, regardless of the specific question or context.
Using ChatGPT

Using the API
In code, this means we need to specify three separate components: the instructions
(what we want the model to do), the output format
(how we want the results presented), and the input code
(the R code we encountered). Finally, we Chain
(combine) the instructions, the output format, and the input code into the final prompt
.
Chaining in prompt engineering means combining different parts of a request to get a precise response from an AI model. By clearly specifying the instructions, desired output format, and input code, we make sure the AI understands and delivers exactly what we need.
instructions = "You will be provided with code delimited by triple backticks. Infer its programming language, then translate the original code to python code and add explanations to it."
# Create the output format
output_format = """
Use the following format for the output:
- Code Language: <The Inferred Orignal Coding Language>
- Code: <The Original Code>
- Code Language: Python
- Transformed code: <The Generated Python Code>
- Explanation < Explanation of the Newly Generated Code >
"""
code = """
income_split <- initial_split(income_frac, prop = 0.80, strata = income)
income_train <- training(income_split)
income_test <- testing(income_split)
"""
# Create the final prompt
prompt = instructions + output_format + f"```{code}```"
response = ChatGPT_API(prompt)
print(response)
And yes, the output meets our requirements: Output Example using ChatGPT.
Although you achieve the same with both strategies, ChatGPT
and the API
, you can already see that you can apply and save a standardized template via the API. It is also clearer and more structured which parts of the prompt are for what purpose. Additionally, using the API allows for better integration with the automation of repetitive tasks, which can save time and reduce errors. Furthermore, the API provides more flexibility in customizing the prompts and responses to fit specific needs.
Conclusion
In this third part of our Prompt Engineering series, we have quietly accomplished a lot. You are now:
- Familiar with retrieving structured outputs from ChatGPT and the API and how to specify the output yourself.
In the meantime, while we have learned these important concepts we have:
- Explored the concept of chaining in prompt engineering to combine instructions, output formats, and input data for precise responses.