BBC Headlined: The AI industry could use as much energy as the Netherlands. While there are several examples of how AI could play an important role in lowering the environmental impact of human activities. For example, by improving climate models, the creation of AI smart homes which reduce household CO2 emissions by up to 40% or the reduction in transportation waste. There are also concerns about AI’s Energy Usage.
Data centers, “The physical homes”, where many AI models like GPT-3
, BERT
, LaMDA
, etc. are trained and deployed are known to be energy intensive. In numbers, these data centers account for roughly 1 to 2% of the world’s total electricity usage. These data centers are not only power-hungry but also thirsty, with Google
, Microsoft
and Meta
collectively withdrawing an estimated 2.2 billion cubic meters of water in 2022. To give an idea of the scale of these numbers,It is equivalent to twice the annual water usage of Denmark (Li et al., 2023).
This article explains how AI models affect the environment, focusing on their carbon and water footprints. It aims to help you understand the energy AI models use, so you can make informed choices about using AI in a way that balances benefits with sustainability.
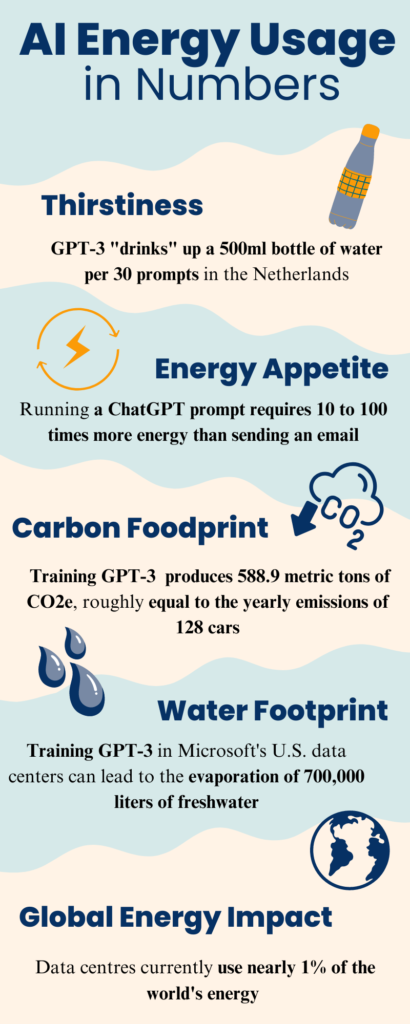
AI Environmental Impact
AI Energy Usage: Understanding the Impact
Artificial Intelligence (AI) refers to a range of technologies and methods that enable machines to exhibit intelligent behavior. One of these methods is Generative AI (Gen AI), which is used to create new content, such as text, images, or videos. For instance, ChatGPT
is a text tool, while OpenAI’s DALL-E
is a tool that turns text prompts into images. Although Gen AI has different applications, they share a common process: an initial training phase followed by an inference phase, where the model requires energy.
Training Phase: The Energy-Intensive Process
The training phase of AI models is often considered the most energy-intensive. In this stage, an AI model is fed large datasets. The model’s initially arbitrary parameters are adjusted to align the predicted output closely with the target output. This process results in learning to predict specific words or sentences based on a given context. Once deployed, these parameters direct the model’s behavior.
The energy used in the training process is measured in megawatt-hours (MWh). To give context, the average household in the United States consumes approximately 10.7 MWh annually. Data on the training of Large Language Models (LLMs) such as BLOOM
, GPT-3
, Gopher
and OPT
reveals the amount of electricity consumed during training, with BLOOM
consuming 433 MWh, GPT-3
using 1,287 MWh, Gopher
using 1,066 MWh and OPT
using 324 MWh. Before settling on the final model, there may have been multiple iterations of training runs.
“Where” to train a large AI model can significantly affect the environmental cost. For instance, the BLOOM
model was trained in France, where 60% of their electricity comes from nuclear power. This allowed the carbon intensity of the BLOOM
model to be very low, at 0.057 kg CO2e/kWh, compared to the average carbon intensity of the US, which is 0.387 kg CO2e/kWh. Therefore, although BLOOM
consumed almost the same amount of energy in the training phase as Llama, the resulting emissions were only one-tenth the amount.
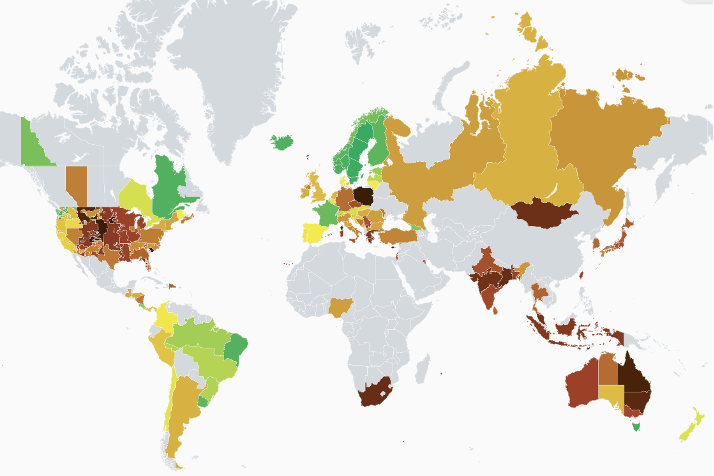
Inference Phase: AI Energy Usage once deployed
Once the models are trained, they are used in production to generate outputs based on new data in the inference phase. In the case of ChatGPT
, this involves creating responses to user prompts.
Although the models used to generate these responses consume a modest amount of energy per session. Alphabet
, the parent company of Google
, announced that the cost of using a Language Model (LLM) can be up to 10 times higher than that of a standard keyword search. A standard Google
search consumes approximately 0.3 Wh of electricity, which suggests an electricity consumption of roughly 3 Wh per LLM interaction. ChatGPT
responds to 195 million requests daily, requiring an average electricity consumption of 564 MWh per day (as of February 2023), or 2.9 Wh per request. Therefore, the energy demand during the inference phase appears to be significantly higher in comparison to the estimated 1,287 MWh used during a GPT-3
‘s training run.
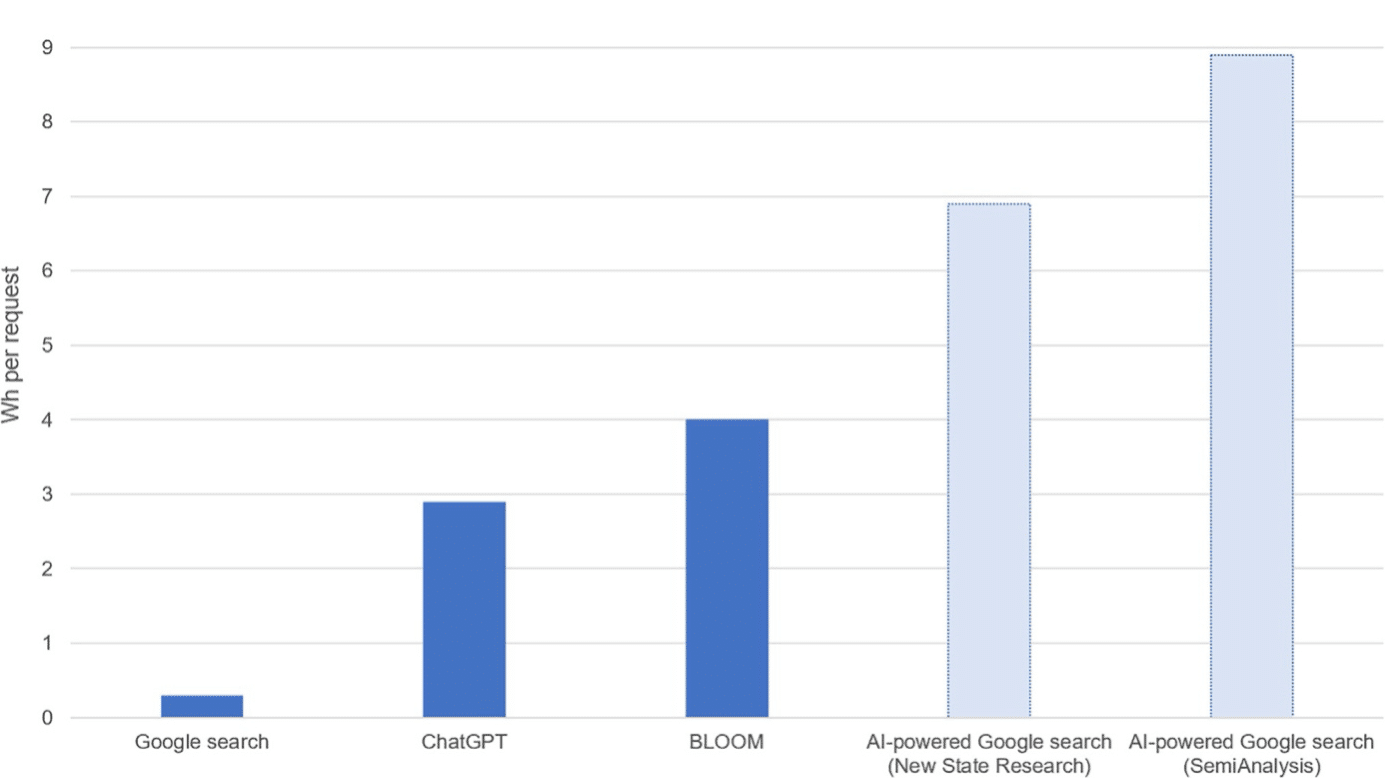
Google
search.Explore how smaller AI models could serve your needs with less environmental cost in our article on smart AI usage strategies. Read more here.
AI Water Usage: The Hidden Environmental Cost
It is important not to focus solely on AI models’ energy usage and carbon footprint but also to consider the water footprint when examining their environmental impact. For instance, the process of training GPT-3
in Microsoft
‘s modern U.S. data centers can cause the evaporation of 700,000 litres of clean freshwater. When considering the larger picture, the global demand for AI could result in 4.2 to 6.6 billion cubic meters of water withdrawal by 2027. This figure is greater than the annual water withdrawal of 4 to 6 times that of Denmark or half of the United Kingdom.
The source article discusses OpenAI'sGPT-3
model. This is possible due to Microsoft's transparency in disclosingWater Usage Effectiveness
(WUE) and Power Usage Effectiveness (PUE) data by location, which is not public information for other companies.
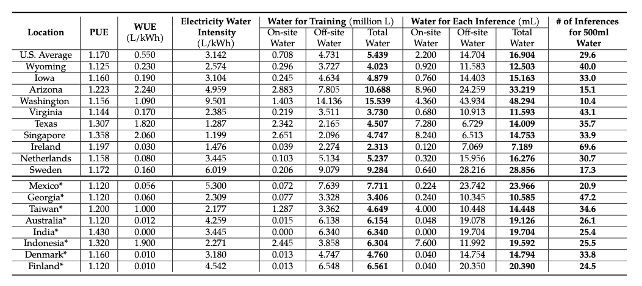
GPT-3
’s average operational water consumption footprint. The PUE and WUE values for these data centers are based on Microsoft
’s projection. So, how thirsty is ChatGPT-3
when we use it? On average, in America, it uses 16.9 mL of water per prompt or interaction, which empties up a 500 mL water bottle after 29.6 instances. However, the thirstiness of ChatGPT-3
shows variation across regions. For example, in Ireland, it takes nearly 70 interactions to empty a 500 mL water bottle.
Regional Variations and Trade-Offs: Carbon vs. Water Footprints
In Sweden, the water bottle empties quickly (17.3 interactions), but this isn’t necessarily detrimental given the region’s abundant water resources. This highlights a potential trade-off: managing the balance between water and carbon footprints. Swedish data centers, leveraging an excess of water and strong renewable energy infrastructure, may consume more water but with a lower carbon impact.
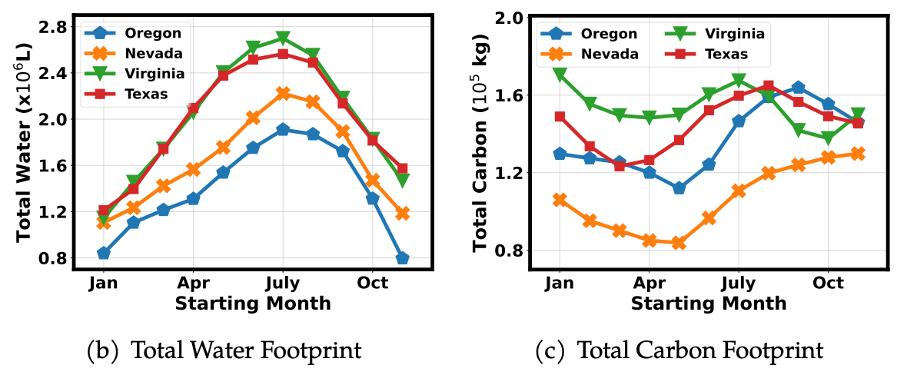
LaMDA
in American data centers.Looking to measure your own AI usage’s carbon footprint? Our step-by-step guide to using CodeCarbon
can help you do just that find it here.
Resources
- Li, P., Yang, J., Islam, M. A., & Ren, S. (2023). Making ai less” thirsty”: Uncovering and addressing the secret water footprint of ai models.
- Vries, The growing energy footprint of artificial intelligence, Joule (2023), https://doi.org/10.1016/ j.joule.2023.09.004